Technology-assisted review (TAR) has been used for nearly two decades in e-discovery matters to reduce costs, increase efficiencies and speed up the often tedious task of manually reviewing emails and user documents for relevance. Fraud examiners can also use TAR techniques (with some guard rails) to find potentially improper payments (PIPs) or transactions in structured data.
A global corporation with operations in Latin America, West Africa and Eastern Europe was being investigated by the U.S. Department of Justice (DOJ) for alleged violations under the Foreign Corrupt Practices Act (FCPA). In coordination with outside counsel and its forensic accounting service provider, the corporation applied technology-assisted review (TAR) techniques to payment transactions, reducing the DOJ’s $30 million in bribery and corruption allegations to less than $8 million and allowing them to settle the case out of court. Did I get your attention?
In this column I’ll describe a fictionalized version of that actual case, where TAR was indeed used as a defense strategy on a real governmental investigation (I’ve changed the numbers and details). The DOJ alleged the company was making improper payments in the form of bribes to government officials, including customs agents, via the use of multiple third-party vendors. The DOJ asserted that more than two dozen vendors had made over $30 million in al- leged bribes and it was up to the company to prove them wrong.
For the sake of calculating costs, assume it takes 30 minutes, on average, to manually trace and review each of the 75,000 invoices that make up the $30 million in alleged bribes from among two dozen vendors. That’s 37,500 hours for attorneys and forensic accountants, at an average $400 per hour professional rate (volume discounted, of course), to manually review these invoices — resulting in a whopping $15 million cost to the company. Given the high-stakes nature of the case, potential reputational damage, and potential fines and/or monitorship facing the company, its board of directors actually agreed, albeit hesitantly, to pay the $15 million. But wait! Along came the forensic data analytics heroes and CFEs to the rescue, with a pitch to the company: What if we used TAR, a concept often used in e-discovery and document review, to efficiently and cost-effectively train a model to statistically predict the likelihood of a potential improper payment?
The Sedona Conference, a nonprofit research and educational institute dedicated to the advanced study of law and policy in the areas of antitrust law, complex litigation, intellectual property rights, data security and privacy, defines e-discovery as: “The process of identifying, locating, preserving, collecting, preparing, reviewing, and producing electronically stored information (ESI) in the context of the legal process.” (See “The Perfect Elevator Pitch for What We Do is Elusive: eDiscovery Trends,” by Doug Austin, eDiscovery Today, March 14, tinyurl.com/299s5eue.)
The meaning of TAR
According to “Technology Assisted Review (TAR) Guidelines,” published by the Bolch Judicial Institute and Duke Law, “TAR (also called predictive coding, computer-assisted review, or supervised machine learning) is a review process in which humans work with software (computers) to train it to identify relevant documents. The process consists of several steps, including collection and analysis of documents, training the computer using software, quality control and testing, and validation. It is an alternative to the manual review of all documents in a collection.”
The guide further explains that TAR reduces the amount of work required in human-based document review, and it carries out the task more quickly and cheaply. And just like a human-based review, TAR involves subject-matter experts to train the computer so that the results are reliable and accurate. [See “Technology Assisted Review (TAR) Guidelines,” Bolch Judicial Institute and Duke Law, January 2019, ti- nyurl.com/mwmyrk68.]
The Bolch Judicial Institute and Duke Law TAR guide was developed by dozens of e-discovery service providers, law firms and academics, and it is often cited, along with other case law and publicly available sources of guidance. This includes the 2017 case law primer from the Sedona Confer- ence. (See tinyurl.com/neddkthz.)
A win-win story
Going back to our case study: What if we could take a statistical sample of invoices and use that to train a predictive model that profiles what is, and what’s not, a potentially improper payment (PIP), aka “suspected bribe?” We could then use that model to statistically determine the company’s exposure. In this case, the forensic technology/data science team determined that a defensible and statistically valid sample was around 3,000 documents. A team of attorneys and forensic accountants manually reviewed these documents (3,000 documents at 30 minutes = 1,500 hours at $400 per hour = $600k). From this sample, around 450 invoices were tagged as PIPs and the remaining 2,550 were tagged as “not PIPs.” Both categories were important for the model to learn from.
Using random forest, neural network and other machine learning techniques, the team was able to tune the model to identify the key variables driving the 450 PIPs. It turns out that when the phrases “facilitation payment,” “help fee,” “round dollar amounts” and “statistically anomalous payments” were present in the transactions, and the payment currency was different than the vendor’s home country (among about a dozen other key variables), such transactions were within a 95% confidence level of being PIPs. It took about six iterations of the machine — either incorrectly predicting a PIP for a transaction that actually wasn’t a PIP (false positive) or failing to flag an actual PIP (false negative) — to get the confidence score, or F1 score, to a confidence level of precision acceptable to the DOJ. (See TAR decision matrix below.) It’s worth noting that the DOJ’s been accepting TAR results in e-discovery matters for more than 15 years, and its in-house expertise on data science, statistics and related topics continues to expand. Fortunately for the company, when the investigations team ran the model with the 450 invoices tagged as PIPs to find “more like this” with a 95% confidence level, the machine returned about 12,500 PIPs amounting to around $8 million in payments — not the original $30 million the DOJ was alleging. The company’s counsel involved the regulators from the outset and maintained transparency throughout, which was a wise decision. The regulators signed off on the process and settled with the company for less than $10 million. Further, instead of incurring $15 million in professional and legal fees, the company spent less than $1.5 million. A win for everyone.
Structured vs. Unstructured data? TAR doesn’t care
This fictionalized, but practical, example illustrates how the same TAR techniques used in e-discovery for email and user documents can also be applied to structured, financial use cases to identify PIPs.
“For over a decade, TAR has been used by attorneys and investigative professionals to assist in making document decisions around relevancy as an alternative to exhaustive manual document review,” Maura R. Grossman, a research professor, e-discovery lawyer and expert on TAR, tells Fraud Magazine.
“The steps typically include collection and processing of the data to be reviewed, training the computer by tagging certain documents for relevancy, quality control procedures and validation of the results. Whether the data is unstructured, such as email or user-created documents; or structured, such as payments to third parties; as long as there is sufficient text and other data elements, it’s all electronically stored information that readily lends itself to technology-assisted review techniques.”
Risk factors to consider
The DOJ has made clear on many occasions, including in their most recent guidance document “Evaluation of Corporate Compliance Programs” [See tinyurl.com/32f9mpvx], that the use of data analytics is an important element in a company’s compliance program. Review efficiency techniques like TAR — for both structured and unstructured data sources — are here to stay and will only grow as tools and technologies evolve.
“Applications in TAR are quite expansive — especially in the M&A space where tremendous amounts of structured and unstructured information need to be reviewed in a timely, efficient and defensible manner,” says Jonathan Nystrom, a pioneer who used TAR back in the mid-2000s at Cataphora Legal (a big data analytics platform developer).
“Due diligence and care are important considerations in every TAR matter as models can be trained to be consistently biased or wrong, almost as easily as they can be trained to be right. There is no substitute for the judgment of a CFE or legal professional when it comes to a document or transaction decision, and the technology amplifies the expert’s decisions either way.”
As Nystrom points out, ethics is an important aspect of this work and the more we learn, the more we can avoid the problem of bias while benefiting from the new technology.
TAR Decision Matrix
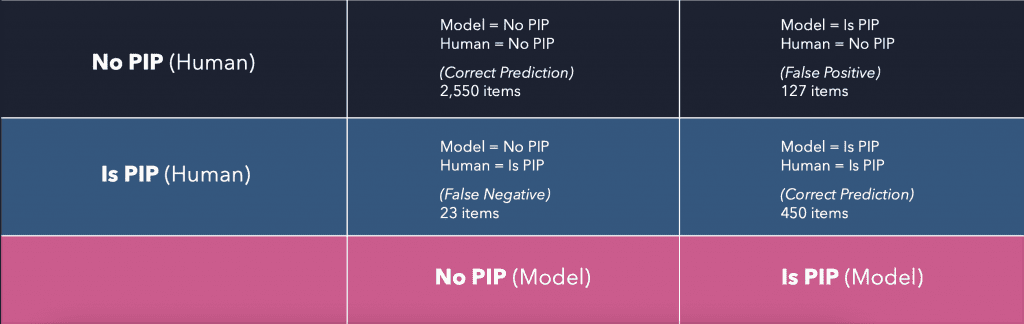
This article was originally published in Fraud Magazine on November 2022.